Fertilizer Subsidy Effects on Cowpea Areas and Spatial Crop Diversity in Malian Villages
Fertilizer subsidy effects on cowpea areas and spatial crop diversity in Malian villages.
Fertilizer Subsidy Effects on Cowpea Areas and Spatial Crop Diversity in Malian Villages
Amidou Assima1, Melinda Smale2, and Bourema Kone3
1Consultant, Michigan State University, Bamako, Mali. amidou.assima@gmail.com.
2 Professor of International Development in the Department of Agriculture, Food and Resource Economics, Michigan State University, East Lansing, MI, USA.*corresponding author. msmale@msu.edu)
3Director, Economie de la Filière, Institut d’Economie Rurale, Bamako, Mali.
Keywords: crop biodiversity, cowpeas, fertilizer subsidies, Mali
Acknowledgements
This work was funded in whole by the United States Agency for International Development (USAID) Bureau for Food Security under Agreement #7200AA18LE00003 as part of Feed the Future Innovation Lab for Legume Systems Research. The data analyzed here were collected under a project funded by USAID/Mali titled “Projet de recherche sur les politiques de sécurité alimentaire au Mali (PRePoSAM)” awarded under the Food Security Innovation Lab’s Cooperative Agreement Number AID-688-A-16-00001. Any opinions, findings, conclusions, or recommendations expressed here are those of the authors alone.
Introduction
The agricultural input subsidy programs reintroduced during the 2000s in numerous countries of Sub-Saharan Africa generally have the short-term goal of boosting food productivity to combat food insecurity over the medium-term. A longer-term, global concern for sustainable development asks how these programs influence the renewal of natural resources and the conservation of agricultural biodiversity. Agricultural biodiversity has been shaped by farmers and communities for millennia and remains a key element of the livelihood strategies of small-scale farmers throughout the world. Here, we examine how fertilizer subsidies influence one component of agricultural biodiversity——the biodiversity of crop species as recognized and grown by farmers.
In the context of welfare economics, input subsidies can be justified when their overall social benefits can outweigh the costs. Costs include not only the fiscal burden of their operation, but also the opportunity cost of foregoing agricultural investments, the cost of displacement or leakage into commercial markets, and externalities such as environmental effects. A “tonnage focus” on raising the production of starchy staples distorts farmers’ incentives to diversify into other nutrient-rich or high-value crops (Gautam 2015)—an imbalance that worsens over time as behavior changes. In their review of the longest, “second-generation” input subsidy program in Sub-Saharan Africa, Dorward and Chirwa (2011) expressed concern not only for the economic but also for the agro-ecological sustainability of Malawi’s program.
Informative reviews of the evidence concerning the outcomes or impacts (effectiveness) of the most recent generation of subsidies in sub-Saharan Africa have been conducted by Druilhe and Barriero-Hurlé (2012), Jayne and Rashid (2013), Wanzala-Mlobela et al. (2013); Kato and Greeley (2016) and Jayne et al. (2018). Few have addressed the externality of crop uniformity or abandonment of non-target crops. In a recent review of the evidence, Holden (2019) notes that although countries such as Norway and Switzerland have been able to implement targeted subsidy programs to incentivize the sustainable use of land, these rely on land and farmer registries, spatial and geographic information that few countries in Sub-Saharan Africa currently possess or can afford. Bioeconomic models of smallholder agriculture in Ethiopia have indicated that linking a conservation requirement to a fertilizer subsidy can enhance both conservation and fertilizer use efficiency (Holden et al. 2006).
In some years, programs such as those undertaken in Malawi and Zambia, where maize is by far the dominant starchy staple, have sought to diversify through also offering the seed of legumes and other crops. Holden and Lunduka (2012) found no effects of input subsidies on intercropping in Malawi; in Zambia, Mason, Jayne, and Mofya-Mukuka (2013) found that input subsidies reduced intercropping, and caused a rise in continuous maize production on the same plot. Also in Zambia, Morgan et al (2019) found that Zambia’s maize-fertilizer subsidy program reduces the probability and extent of fallowing and intercropping of maize with other crops, also inducing longer continuous cultivation of maize in the same plot over time.
The most common arguments linking staple foodcrop productivity and crop diversity appear to be based on theory and direct observation of the processes of change induced by the Green Revolution, particularly in its locus—the wheat and rice baskets of Asia. For example, Dwivedi et al. (2017) observe that the most important cropping system in South Asia, the rice–wheat cropping system of the Indo-Gangetic Plains, “revolutionized agriculture during the Green Revolution which, on the one hand, enhanced food and nutritional security, and displaced legumes from the system on the other”(p. 845).
In the irrigated, Indo-Gangetic plains of South Asia, Pingali (2017) observed that agricultural intensification (the increase in output per unit of land used in cultivation, land productivity, or “yield”) led to concentration of area in input-responsive crops such as rice and wheat over millets, pulses and root crops—a process that preceded but was accelerated by the Green Revolution. He observed a greater crop diversity in rainfed environments where soil types and micro-climates are highly variable, yields and yield response to fertilizer are lower. In environments prone to drought, high temperatures or flooding stresses, various types of millet and sorghum are grown and farmers often plant multiple crops in the same field. He noted that these older (which some call traditional) systems tended to endure.
Crops compete not only for land and water, but for investments in research and value chain development. Kahane et al. (2015) spell out the range of benefits of “neglected and underutilized” crop species for health and nutrition, environmental sustainability, and prosperity of human populations. The authors refer to these crops, which include the legume cowpea indigenous to West Africa, as “development opportunity crops,” also highlighting the agronomic and economic synergies of growing these alongside or in combination with major crops.
Mali's fertilizer subsidy program targets staple cereals (cotton, rice, maize, and at a reduced rate, sorghum), embedding incentives for farmers to grow these crops more than others. Farmers may tend to allocate the majority of their plots to the target crops in order to minimize risks and uncertainties (Di Falco and Perrings, 2005), leading to a reduction in the biodiversity of crop species on farm and village scales.
Our analysis contributes to a sparse literature that relates input subsidies to crop species diversity in several ways. First, we test the impacts of the fertilizer subsidy program in Mali on the richness and evenness of crops grown by small-scale farmers. Second, we test the impacts of the program on area shares planted to a neglected crop (cowpea) as both a primary and an intercrop. Third, we conduct our analysis with a simultaneous equations model estimated at the scale of the village. As compared to analyses of decision-making on an individual farm, this more aggregated perspective provides insights about the farming landscape. We apply both instrumental variables Tobit (IV-Tobit) and the generalized method of moments (GMM). Applying GMM serves as a robustness check on subsidy effects and also enables us to explore interrelationships among measures of crop species diversity.
Why crop diversity matters for farm policy
Spatial (farm landscape) and temporal (sequence) diversification of crops can contribute to agroecosystem resilience by enabling the suppression of pest and pathogen transmission and buffering crop production against climate variability and extreme events (Gaudin et al. 2015; Lin 2011). Landscape‐level crop diversification can improve biological control in agroecosystems, lowering dependence on insecticides while enhancing yield stability (Redlich, Martin and Steffan-Dewenter 2018). Isbell et al. (2017) conclude that benefits of diversifying agroecosystems are likely to be greatest where the aims are to intensify production in sustainable ways while also reducing the need for conventional inputs. They describe diversification as an “essential tool” in the quest for sustaining production and ecosystem services.
Intercropping is the simultaneous cultivation of more than one crop species on the same piece of land and is regarded as the practical application of basic ecological principles such as diversity, competition and facilitation (Hauggaard-Nielsen et al. 2008). In their meta-analysis of 33 studies conducted in tropical agriculture, Raseduzzaman et al. (2017) conclude that cereal intercropping with legumes significantly reduces the high instability of cereal yields in all climatic zones. In Mali and Burkina Faso, studies have shown the same with respect to intercropping with cowpeas (Sogoba et al. 2020; Zoundi et al. 2007).
With respect to input intensification, in the Midwest of the US, Davis et al. (2010) found that more diverse cropping systems can use “small amounts of synthetic agrichemical inputs as powerful tools with which to tune, rather than drive” the productivity of the ecosystem—even exceeding that of less diverse systems. In Southern Africa, integrating maize with legumes as intercrops or in rotation can allow farmers to achieve high and stable yield under varying rainfall, with modest fertilizer investments (Chimonyo, Snapp and Chikowo 2019).
Crop diversity is economically valuable. Renard and Tilman (2019) analyzed 5 decades of data on 176 crops in 91 nations and found that greater crop diversity at the national scale is associated with greater temporal stability in national food production. Studies in the applied economics literature have tested the effects of crop diversity on crop productivity and resilience at the farm and regional scales of analysis. In France, Donfouet et al. (2017) found a positive effect of crop diversity on overall productivity, particularly in lower rainfall areas. Using historical data, Omer et al. (2007) demonstrated the positive effects of non-cropped areas, hedgerows, and plant species diversity on the production frontier of the cereals system in the eastern UK. In Papua, New Guinea, Coelli and Fleming (2004) found diversification economies between subsistence food production and both coffee and cash food production. In Vietnam, Nguyen (2017) showed that diversifying by integrating cash crop and food crops generated significant complementarity and potential economies of scope.
In Uganda, Kozicka et al. (2020) found that increased crop diversity on farms allows farms to generate higher revenues and increases their capacity to adapt to climate change. In marginal areas of Ghana, Bellon et al. (2020) find that crop diversity is positively related to both consumption of food produced on the farm, but also to cash income from harvests sold in markets. Michler and Josephson (2017) concluded that crop diversity reduces poverty in Ethiopia. Such findings confirm that crop diversity is a potentially viable “entry point” for agricultural development strategies.
Methods
Data
We use data collected as part of the Mali Living Standards Measurement Survey-Integrated Survey of Agriculture (LSMS-ISA), conducted in 2014-15 and 2017-18. The LSMS-ISA are nationally representative surveys that collect detailed information on demography and aspects of living standards such education, health, and employment. The agricultural component of LSMS-ISA survey includes sections on plot characteristics, inputs and institutions, input subsidy, input use and others. The plot characteristics section provides details on the size of the plot and the crops grown on the plot, as well as information on intercropping. At the community level, data collected cover aspects such as infrastructure and the characteristics of the enumeration areas such as rainfall, temperature, cropland density and population density.
In Mali, a nationally representative sample of 1070 enumeration areas, selected with a probability proportional to size in all regions except Kidal, was retained for the two surveys (CPS / SDR, 2019). Due to the security situation in the country, the LSMS-ISA team was not able to collect data in all selected enumeration areas during the first round. A sample of 3,804 households in 989 enumeration areas were interviewed in the 2014-15 survey, whereas enumerators were able to reach a sample of 8,390 households in 953 enumeration areas in 2017-18.
Since our unit of study is the enumeration area, we limit our analysis to the subsample of enumeration areas that have consistent and detailed information about the crops. This gives a sample of 1,621 enumeration areas of which 830 were covered in 2014-15 survey and 791 in 2017-18 survey.
According to the last population and housing census of 2009 (RGPH2009), an enumeration area (SE) is a geographical area with an average of 700 inhabitants in rural areas and 1,100 inhabitants in urban areas. Populations have evolved considerably since the last census, but the SE is the unit on which the LSMS sample and other nationally representatively samples in Mali continue to be based. To meet the standard size as of 2009, an SE covers either part of a village administrative unit, one or several village administrative units. Below, for simplicity, we refer to the enumeration area as a “village.” While this descriptor may not be accurate from an administrative standpoint, our aim is to analyze crop species diversity at a larger scale of analysis than the individual farm that is also consistent as a unit. The LSMS-ISA refers instead to “community” survey component, which we find to be more problematic because it implies social cohesion which we are unable to confirm. We draw on all data categories included in the survey: household, farm, and community.
Diversity outcome indicators
Agricultural biodiversity includes all the components of biological diversity of relevance to food and agriculture together with the components of biological diversity that constitute the agro-ecosystem, including the variety and variability of animals, plants and micro-organisms at the genetic, species and ecosystem levels (FAO, 2016). Over the course of human history, modern human activities, and notably agriculture, have degraded biodiversity (Campbell et al 2017; Foley et al. 2011; Pereira et al. 2012). Unlike other components and forms of biodiversity, such as forests and wild grasslands, agrobiodiversity cannot exist without humans because agriculture is human-made.
Here, we use the term "crop diversity" in referring to the observable variation among crops as recognized by the farmers who grow them, rather than other scientific taxonomies. It is also important to distinguish between the concept of diversity and the measurement tool. The measurement tool is simply a mathematical construct that enables a given concept of diversity to be incorporated more conveniently in an analytical model. Once a concept has been chosen, an index allowing it to be expressed as a number or scalar that varies over the units of observation can be calculated (Meng et al. 1998).
We draw on and adapt the biological concepts of crop richness and proportional abundance, or evenness (Magurran 2004; Smale 2006; Jarvis et al. 2008) to represent crop diversity. Richness refers to the count of different types of plants in the plant population encountered per unit of a geographically defined area (in our case, the enumeration area or village cluster. A simple count ignores relative frequencies (Jarvis et al. 2008). Evenness accounts for the similarity among frequencies of the different plant types. Low evenness implies dominance by one or a few types.
To measure these concepts, we adapt indices used in the ecological literature. Magurran (2004) refers to two easily measured indices of spatial richness. Each index controls for “sampling intensity” by dividing the count of different types by the total number of individuals in the reference area. Since we use area under crop as a proxy for the number of plants, we choose to use the Menhinick index. This way we avoid dealing with negative values of the index with areas less than one hectare. We generated the Menhinick index by standardizing the count of different crops reported by all households in each enumeration area by the square root of the total area under crops.
To express the concept of proportional abundance we use the Shannon index, which has often been used in ecology and adapted to studies of on-farm diversity at the household scale of analysis (Smale, 2006; Bellon et al., 2020). We computed the Shannon diversity index as Dsh = −∑Piln(Pi), where Pi is the share of the ith crop in the total area under crops per enumeration area. The Shannon index reflects both the richness and evenness of the distribution of individuals in a population, or proportional abundance.
We measure cowpea area shares in two ways. In the first, we calculate the proportion of the total crop area of all plots in the enumeration area that are planted to cowpea as a primary crop. This indicator measures the relative importance of a neglected crop in the local farming system. The second is the proportion of the total crop area of all plots in the enumeration area that are intercropped with cowpea, reflecting the particular agronomic role of cowpea in the farming system as a nitrogen-fixing legume.
Econometric strategy
The conventional problem encountered in impact studies based on observational data is potential endogeneity. In the case of this study, we seek to investigate the causal relationship between subsidized fertilizers and outcome variables such as crop diversity and cultivation of a neglected crop, cowpea. The same observed and unobserved characteristics could affect both outcome variables, crop diversity and cowpea cultivation, and the decision to participate in the subsidized fertilizer program. Indeed, farmer's expected utility maximization motivates their participation in the subsidy program. Input subsidies reduce the cost of applying a scarce, productivity-enhancing input and can induce farmers to allocate more of their cultivated land to targeted crops, thereby reducing crop diversification, to maximize their utility. However, some farmers may decide not to participate in the subsidy program and choose to maximize their utility by diversifying crops while avoiding climate and market uncertainties. Therefore, both participation in the subsidy program and crop diversification depend on the intrinsic characteristics of farmers. Subsidized fertilizer as a treatment variable is therefore potentially endogenous. Further, our data reveal that subsidized fertilizer has a heavy concentration at zero even at the village scale. Our diversity indicators are also generally censored in form.
We apply two econometric approaches to test and control for potential endogeneity of the subsidy in these diversity outcomes and handle censored structure. In the first, we apply a two-stage approach using instrumental variables (Smith and Blundell, 1986; Newey, 1987). In the second, we apply the generalized method of moments. This second approach serves as a robustness check but also allows us to model interrelationships among diversity indicators in a simultaneous equation framework.
Following Tobin, who was the first to introduce the Tobit model, we specify our basic model as
Yit* = Sitα +Xitβ + εit ; Yit = Yit* if Yit* > 0 and Yit = 0 otherwise , (1)
where i and t denotes the enumeration area (village), t refers to the year, and Yit expresses the observed diversity outcomes. Yit* is the corresponding latent dependent variable. Xit is a matrix of exogenous, village characteristics. α and β are vectors of coefficients and is the vector of errors. is the potentially endogenous variable representing the total amount of subsidized fertilizer applied by all farming households at the village scale, which we refer to as the “subsidy incidence.”
A normal distribution of the error terms, conditional on independent variables Xit and Sit, supports the validity of the model. When the incidence of subsidized fertilizer use among farmers is endogenous, however, this condition is violated. To test and control for the potential endogeneity of subsidized fertilizer, the basic Tobit model is modified by modeling the subsidy simultaneously in two stages using instrumental variables. We characterize the subsidy incidence as a function of the same matrix Xit of the exogenous variables, and a vector of instruments Zit:
Sit = Xitδ + Zitγ + μit . (2)
In equation (2), δ and γ are vectors of coefficients and μit is a vector of error terms.
The simultaneity of equations 1 and 2 calls for testing and controlling for endogeneity through use of instrumental variables. The exclusion restriction requires that we choose instrumental variables (IV) that are correlated with subsidized fertilizer but uncorrelated with the errors of the outcome variable regressions. In the present study, we use total commercial fertilizer applied in the village and extension service variables to instrument the subsidized fertilizer variable. Variables such as DNA (Direction National de l’Agriculture), ON (Office du Niger), CMDT (Compagnie Malienne de Développement des Textiles) and OTHERS indicate whether one of these public extension services is present in the village (Table 1). Extension services are a source of awareness for farmers about their eligibility for the fertilizer subsidy program and constitute an important channel for distribution of subsidized fertilizer. Farmers in villages where these services exist are more likely to have better access to the fertilizer subsidy. The use of subsidized fertilizers is more widespread in the ON and CMDT zones, where the structure of the extension services is formalized and hierarchical given the economic importance of rice and cotton to the national economy (Smale, Thériault and Mason, 2020). Services are considerably less comprehensive in the DNA areas, which are sometimes referred to as “non-encadrés.” OTHERS includes the presence of non-governmental organizations (NGOs).
However, there is no reason to believe that these instrumental variables have a direct correlation with crop diversity and cowpea cultivation. None of the extension systems actively promotes diversification since they are focused on the key crop of the farming system (e.g., cotton, rice, and to a far lesser extent, dryland cereals). Historically, maize was also promoted in the cotton zone to support food security, but both of these were targeted crops at the time of the surveys.
We assessed the suitability of the IV-Tobit model by performing a Wald test of exogeneity of the instrumented variable. When we reject exogeneity with the Wald statistical test, we conclude that the data are consistent with the presence of endogeneity and thus the IV-tobit model is preferred. To check the relevance of the instruments, we used the first-stage Stock and Yogo F-statistic. The critical value of the test depends on the number of endogenous regressors and the number of instruments. For an endogenous regressor and seven instruments, the critical value of the test is equals 19.86. When the first-stage F-statistic is greater than this critical value, we can conclude that our instruments are relevant. We estimate IV-Tobit regressions for each of our diversity outcome indicators.
Next, we apply the generalized method of moments (GMM) to express the relationship among the outcome variables, crop diversity and cowpea cultivation, and the fertilizer subsidy in a simultaneous equation system. By addressing the simultaneity of mutual influence of crop diversity, cowpea production and fertilizer subsidy, the GMM model allows us to obtain consistent estimates. This method improves the efficiency of estimates compared to a single-equation approach (Wooldridge, 2002). Some conditions must hold for the GMM model estimates to be consistent. To ensure that these conditions hold, we performed a series of diagnostic tests. We test the endogeneity of subsidized fertilizer by using the Hansen's J statistic of over-identifying restrictions. We checked the strength of the instruments using the F-test of the reduced regression form.
We found several studies that have applied the GMM approach to examine effects of agricultural policy with simultaneous equations. Mary (2013) used the GMM approach to study the effect of subsidies on French farms. Akbar and Jamil (2012) used the GMM technique to explore the effects of monetary and fiscal policies on agricultural growth in Pakistan. More recently, Bellon et al. (2016) used GMM to test the linkages among crop diversity, diet diversity, and market participation among rural households in Benin.
We use GMM to express the relationship among the outcome variables and the fertilizer subsidy through the system of four equations:
Dm = αS+ Xβ+ ε (3)
Ds = γS + Xδ+ μ (4)
Dc = exp(ζDm+ νDs +θS + Xω + η) (5)
Dic = exp ( τDm+ πDs + χS + Xφ + ψ) (6)
Dm is Menhinick’s index of richness, Ds is Shannon’s index of evenness, Dc is the share of plots planted with cowpea as a primary crop and Dic is the share of cowpea plots planted as an intercrop.
Our GMM model has two components, or dimensions. One component tests the effect of the fertilizer subsidy on crop diversity and cowpea cultivation observed at the village scale. The second tests the interrelationships among the richness and evenness of crops grown and cultivation of cowpea, a neglected crop, as a primary crop and intercrop. All models were estimated in STATA 15.
Independent variables
Past literature tests and confirms the importance of household, market, and agroecological characteristics measured at various scales of analysis as determinants of crop diversity on farms (Smale et al. 2003; Benin et al. 2004; Gebremedhin et al. 2006; Nagarajan et al. 2007; Bellon et al. 2020). Distance to markets, or remoteness, affect the access of farming households to seeds of other crops and commercial sales of crop harvests. Sales generate incentives for specialization. We use the distance to the nearest tarmac round and to the administrative capital of the region, where the largest regional market hub and population are found. Population density potentially induces land-saving technical change and agricultural intensification, including larger numbers of farm production activities undertaken, such as additional crops. Household labor size, and the dependency ratio, may condition the ability of the family to respond to incentives such as these. Education affects access to information and knowledge about farming opportunities. With respect to agro-ecological features, we hypothesize that, other factors held constant, farmers may grow a greater number of crops as an adaptation to various rainfall and temperature regimes, including isohyets.
Table 1 presents variable definitions and descriptive statistics at the village level for the two periods covered by the study.
Results
Descriptive statistics
The graphs in Figures 1 and 2 provide a visual understanding of the distribution of the three indices, namely the crop count index, the Menhinick's diversity index and the Shannon diversity index, by isohyet. The dots indicate the index value and the green line represents the mean of the index. Overall, areas south of the 600mm isohyet, including Kayes, Koulikoro, Sikasso and Segou, have a higher mean crop diversity. Areas north of the 400mm isohyet, including Mopti, Timbuktu and Gao are poor regions in terms of crop diversity. This pattern is in accordance with expected moisture regimes.
With respect to the count index, regions south of the 800mm isohyet have the highest mean value of the index (around six crops). The three regions north of the 400mm isohyet regions have the lowest mean count index, with the regions of Timbuktu and Gao having a mean count value of less than two. Regarding the Menhinick index, the differences in means between the southern regions are not significant even though there is a slight difference in favor of Kayes. Logically, the regions of Mopti, Timbuktu and Gao, in which the mean count index does not exceed two, also have the lowest Menhinick index.
The most diversified region according to the Shannon index is Sikasso, followed by Koulikoro and Kayes respectively. There is no difference between Segou and Kayes regarding that index. Timbuktu and Gao account for the lowest median value of the Shannon index. Thus, crops in the southern regions are not only more diversified than the northern regions but they are also better distributed.
Figure 3 depicts the distribution in the share of cowpea plots as the main crop and associated crop. The main cowpea producing regions are areas north of the 600mm isohyet, mainly Mopti, with on average of nearly 6% of the plots allocated to cowpea cultivation as the main crop and 3% as an intercrop, followed by the Koulikoro and Segou. Mopti’s lead reflects the fact that the farming areas across Bankass and Koro are major producers of millet and small ruminant livestock. Cowpea is popular there as both a primary crop and an intercrop with millet, and cowpea forage is fed to sheep. The Timbuktu region occupies sixth place with around 2% of the plots dedicated to cowpea as the main crop. Cowpea production is almost nil in Gao and Bamako.
Econometrics findings
Table 2 summarizes the results of the first stage IV-Tobit. As expected, the coefficients of all instruments are positive and significant. If the extension service variables, CMDT, ON and DRA, are strongly correlated with subsidized fertilizers with a level of statistical significance of 1 to 5%, the correlation between commercial fertilizer and subsidized fertilizer is low with a level of statistical significance of 10%. We argue with regard to the strong correlation between the variables of extension services and subsidized fertilizers, that in Mali, the extension services (CMDT, ON and DRA) represent the main channels of access to subsidized fertilizers. Indeed, the CMDT is the path of cotton producers and the ON, that of irrigated rice farmers. Farmers in areas where these services exist have access to subsidized fertilizer on credit through these extension services.
While the use of pesticides increases the amounts of subsidized fertilizer applied—which makes sense, given farmers who use one purchased input may be more likely to buy another, and there is an increase in pest infestation is expected as yield rises due to fertilizer use. Organic fertilizer is negatively associated with subsidized fertilizer applied suggesting that in this range of use, Malian farmers are treating these inputs as substitutes despite that both are recommended for maintenance of soil structure and fertility. Higher rainfall is associated with greater use of subsidized fertilizer. In addition, distance to the road and temperature are negatively correlated with subsidized fertilizer. The F statistic of the first step regression is 26.79 (Table 2). This value is greater than the critical value of the Stock-Yogo test at the 5% significance level for a single endogenous regressor and five instruments. In addition, all our instrumental variables are significant. Therefore, our instrumental variables are relevant and strong.
The results presented in Table 3 show that the Wald exogeneity test is statistically significant at the 5% level. This leads to reject the null hypothesis of exogeneity of the subsidy variable. Thus, subsidized fertilizer should be treated as an endogenous variable and the IV-Tobit model is appropriate to account for potential endogeneity bias.
The estimated coefficient of the subsidy variable is statistically significant at 5%. Subsidized fertilizer applied is negatively associated with crop richness as measured by the Menhinick index. By contrast, subsidized fertilizer is positively associated with crop proportional abundance as measured by the Shannon index. One explanation of these results is that the Malian fertilizer subsidy program targets crops and therefore encourages farmers to focus on a small number of crops targeted by the subsidy, but these are more evenly distributed. The negative association of subsidized fertilizer with the share of area planted with cowpea as a primary crop at the village scale is consistent with negative effects on richness and use of non-targeted, minor, or neglected crops, which has agronomic implications. Non-subsidized crops, such as cowpeas, are likely to be losing ground as primary crops in farmers’ fields to the major subsidized crops (rice, maize and cotton). The effect on cowpea planted as an intercrop is negative but not statistically significant.
The coefficient on the distance to regional capital variable is positive and statistically significant in all models. Thus, there is a positive correlation between the diversity of crops as measured by the Menhinick crop richness index and the Shannon indices, the share of plots sown with cowpea and the remoteness of villages. This is understandable as crop diversification and cowpea cultivation is an adaptive strategy to ensure household resilience and food stability in more isolated areas. Numerous previous studies have established strong linkages between remoteness and inter- or intra-species crop diversification, though product markets can influence these variables positively if price premia or niche markets are present (Brush, Taylor and Bellon 1992; Meng et al. 1998; Benin et al. 2004; Van Dusen and Taylor 2005; Birol, Smale, and Gyovai 2006; Bellon et al. 2020).
Average household size is significantly and negatively associated with crop richness index. This confirms the finding of other studies in sub-Saharan Africa that have reported that large households appear to adopt a more specialized strategy with less crop diversity (Bellon et al., 2020). In the Ethiopia highlands, Benin et al. (2004) found this result for infra-species crop diversification, but not for diversification among cereal crops. We find that larger household size is positively related to proportional abundance as well as to cowpea area shares at the village scale. Farming households of larger size in Mali are able to confront « lean » or « hungry » seasons of uncertain cereals production by producing other crops such as cowpea. When cereals supply on markets is constrained, it is especially difficult for household heads of larger families to purchase the quantities needed to feed their families. Cowpea is a means of coping with this challenge because of it planting period.
Education of plot managers at the village scale significantly and positively influences crop richness while reducing the evenness among crops. It significantly and positively affects the share of area sown with cowpea as the primary crop and has no effect on cowpeas in intercropping. As education improves access and use of technical and climate-related information, educated farmers may be more willing to diversify their crops and varieties. Again, this result has been reported in the literature we cite here (Benin et al. 2004; Van Dusen and Taylor 2005).
The temporal trend of crop diversity is negative and significant for the Menhinick index, and positive for the Shannon index. Survey year has no significant effect on cowpea as the main crop but a negative and significant effect on cowpea as an intercrop.
Proximity to the road does not seem to have a significant effect on any of the dependent variables. This is encouraging with respect to access to subsidized fertilizer—suggesting the intended “reach” of the subsidy to small-scale farmers throughout Mali. As expected, rainfall has a positive and significant correlation with all outcome variables, contributing to higher crop diversity and expansion of cowpea production areas. This is particularly the case since rainfall is the climatic parameter that determines the Malian production system, which remains largely dominated by rain-fed agriculture. Temperature has a positive association with crop diversity and a negative relationship with cowpea production. This indicates that an increase in temperature would lead to an increase in crop diversity and a decrease in cowpea production.
Use of organic fertilizer does not affect the Menhinick richness index, but shows a positive effect on the Shannon index or proportional abundance and the share of plots sown with cowpea as the main and intercrop. By contrast, pesticide use negatively affects all our outcome variables; both crop diversity indices and cowpea production outcomes deteriorate as the share of plots treated with pesticide increases.
Population density is negatively associated with the Shannon crop diversity index and the share of plots sown with cowpea as the main and intercrop crop. Although the population density coefficient is not statistically significant, it shows a negative effect in sign on the Menhinick crop diversity index. These findings are consistent with the expectation that higher population density is associated with specialization and more limited cropping areas per capita.
Table 4 presents result from the GMM estimations. Overall, the GMM estimation results are consistent with those of the IV-Tobit model. The findings lead to the same inferences regarding the sign and significance of the subsidy variable for all outcome variables in both models. Of all the repressors used in this study, only the temperature and year variables lead to markedly different conclusions. The main difference between the two models is that the temperature variable has a negative effect on the Shannon index in the GMM model, while it shows positive effects in the IV-Tobit model. The two models lead to the same results with respect to the variable year, at least in terms of signs. The similarity between the two models suggests that our results are robust and increases our confidence in our results.
In addition, the GMM model demonstrates the interrelationships between crop diversity and cowpea production. The share of cowpea plots increases with the diversity of crops. The Shannon index has a positive and significant effect on the share of cowpea plots as the main crop but does not seem to have significant effects on the share of plots with cowpea intercrops. Meanwhile, the Menhinick index has positive and significant effects on the share of cowpea plots as the main and intercrop species. In other words, the higher the number of crops grown by farmers in the village overall, standardized by total cultivated area, the greater is the percentage of the area allocated to this leguminous, minor crop. This suggests that cowpea plays a role in farmers’ strategies when they choose to diversify at the scale of the village. The farming environments matters for raising the chances that minor crops such as cowpea are grown and their planting density.
Conclusions
One of the longer-term consequences of input subsidy programs currently implemented in numerous countries of Sub-Saharan Africa is their implications for the sustainability of farming systems. In a vast and rigorous empirical literature on the topic of “smart” subsidies in this region, this topic has not been much addressed. We have explored how the fertilizer subsidy in Mali influences one component of agricultural biodiversity—crop species diversity. We contribute to the literature on input subsidy impacts by testing the associations among the fertilizer subsidy, crop species diversity and cultivation of a neglected crop (cowpea) at the at the village scale in Mali.
To test these relationships, we have applied two econometric models, namely the IV-Tobit model and the GMM model, to LSMS-ISA data organized as a panel at the scale of the enumeration areas. We refer to this scale as “the village” while recognizing that the enumeration area, which constitutes a statistically valid and consistent unit, is neither a coherent social nor administrative unit. Our aim in choosing this unit is to permit panel data analysis at a higher scale than the individual household farm. These LSMS-ISA data in Mali are not a household panel. The GMM model provides a robustness check on the effect of the fertilizer subsidy and also enables us to explore simultaneity and linkages among different diversity indicators.
We measured diversity outcomes in terms of the Menhinick index of richness, the Shannon evenness index of proportional abundance, the share of cowpea area cultivated as a primary crop, and the share of area in crops grown with a cowpea intercrop. The Menhinicak and Shannon indicators express spatial diversity and are often used in the ecological literature. We adapt them here by using area share to represent the density of the plant population.
Our graphs indicate clearly that areas in higher isohyets, with higher expected rainfall, also have greater average crop diversity as measured by either richness or proportional abundance. The northern Sahelian regions of Timbuktu and Gao are least favored and Sikasso, the southernmost region of Mali, is most favored. The region of Mopti in the central “neck” of Mali, which includes Bankass and Koro that produce large amounts of millet, sorghum, and small ruminants, leads in cowpea cultivation as either a main crop or intercrop.
The quantity of subsidized fertilizer received at the village scale is strongly and negatively associated with crop richness,but is positively associated with evenness. These findings are consistent with both of our working hypotheses. That is, the subsidy incentivizes the allocation of land to targeted crops, possible leading farmers to discard other crops. On the other hand, improving productivity of targeted crops through application of mineral fertilizer can release land for cultivation of other crops are more even allocation of land among crops currently grown. Further, leakage from targeted crops to non-targeted crops happens; monitoring the use of subsidized fertilizer among Mali’s millions of smallholder farmers would be impossible.
We also find that the subsidy is strongly and negatively associated with the cultivation of cowpeas as a primary or a secondary crop, demonstrating the potentially detrimental effect on minor crops with potentially high value in local grain or fodder markets (for either grain or fodder), containing important nutrients for humans and livestock, and/or contributing to nitrogen fixation in the aged, degraded soils found in much of Mali’s farming systems. The richer the village remains, the higher the predicted share of area that is allocated to cowpeas. Overall crop richness and continued cultivation of a minor crop are positively associated.
Clearly, the fertilizer subsidy program as currently designed in Mali favors certain crops of recognized importance to the national economy (cotton) and to the starchy staple needs (particularly maize and rice) of the nation’s predominantly rural population. Millet and sorghum are subsidized at only 35% of the total recommended fertilizer rate per hectare, as compared to 100% for cotton, maize and rice (Niangado 2020). The probable justification for differential subsidization is that sorghum and millet are less responsive to fertilizer than maize or rice (Haider et al. 2018). Findings suggest that favoring targeted crops over others may have long-term consequences for farming systems in Mali. Policies that privilege targeted crops over non-targeted crop may encourage farmers to cease growing other high-value crops and crops with specific contributions to soils nutrients or to the nutrient composition of the Malian diet.
One such crop is cowpea. Results generally reveal a negative effect of the subsidy on cowpea as both primary crop and intercrop. Of all of these targeted crops, millet is most often grown in association with cowpea in Mali, and especially in the Mopti region. Intercropping practices offer many advantages but improved understanding of the ecological mechanisms associated with planned spatial diversity, including additional benefits with associated diversity, is needed to enhance the benefits achieved (Hauggaard-Nielsen et al. 2008).
Reconsideration of program design to address the possibility of combining the fertilizer subsidy with other inputs such as seeds of non-target crops or soil and water practices, as has been attempted in other programs launched in Sub-Saharan Africa, is one policy direction. For example, cowpea growers would benefit from a microdose of fertilizer or provision of improved cowpea seed.
Competing interests
The authors declare no competing interest.
.
References
Akbar, M. and Jamil, F. (2012) ‘Monetary and fiscal policies’ effect on agricultural growth: GMM estimation and simulation analysis’, Economic Modelling, 29. doi: 10.1016/j.econmod.2012.06.001.
Bellon, M. R., G.D. Ntandou-Bouzitou, and F. Caracciolo. 2016. On-farm diversity and market participation are positively associated with dietary diversity of rural mothers in southern Benin, West Africa. PLOS ONE. DOI:10.1371/journal.pone.0162535.
Bellon, M.R., B.K. Kotu, C. Azzarri,and F. Caracciolo. 2020. To diversify or not to diversify, that is the question: Pursuing agricultural development for smallholder farmers in marginal areas of Ghana. World Development 125, 2020, https://doi.org/10.1016/j.worlddev.2019.104682.
Benin, S., M. Smale, J. Pender, B. Gebremedhin and S. Ehui. 2004. The economic determinants of cereal crop diversity on farms in the Ethiopian Highlands. Agricultural Economics 31: 197-208.
Birol, E, M. Smale, and A. Gyovai. Explaining Farmer Demand for Agricultural Biodiversity in Hungary’s Transition Economy. In Smale, M. (ed.) Valuing Crop Biodiversity: On-Farm Genetic Resources and Economic Change. Wallingford, Oxon, UK: CAB International.
Brush, S.B., J.E. Taylor, and M.R. Bellon. 1992. Technology adoption and biological diversity in Andean potato agriculture. Journal of Development Economics 39: 365-387.
Campbell, B.M., D.J. Beare, E. M. Bennett, J. M. Hall-Spencer, J. S.I. Ingram, F. JUaramillo, R. Ortiz, N. Ramankutty, J.A. Sayer, and Dr. Shindell. 2017. Agriculture production as a major driver of the Earth system exceeding planetary boundaries. Ecology and Society 22 (4): 8.
Chimonyo, V.G.P., S.S. Snapp, and R. Chikowo. 2019. Grain Legumes Increase Yield Stability in Maize Based Cropping Systems. Crop Science 59: 1222-1235. https://doi.org/10.2135/cropsci2018.09.0532.
Coelli, T. and E. Fleming. 2004. Diversification economies and specialization efficiencies in a mixed food and coffee smallholder farming system in Papua New Guinea. Agricultural Economics 31 (2-3)” 229-239.
Davis A.S., J.D. Hill, C.A. Chase, A.M. Johanns, M. Liebman. 2010. Increasing cropping system diversity balances productivity, profitability and environmental health. PLoS One. https://doi.org/10.1371/journal.pone.0047149.
Di Falco, S. and Perrings, C. 2005. ‘Crop biodiversity, risk management and the implications of agricultural assistance’, Ecological Economics, 55(4), pp. 459–466. doi: 10.1016/j.ecolecon.2004.12.005.
Donfouet, H. P.P., A. Barczak, C. Détang-Dessendre, E. Maigné. 2017. Crop production and crop diversity in France : A spatial analysis. Ecological Economics 134: 29-39.
Dorward, A. and E. Chirwa.. 2011. The Malawi agricultural input subsidy programme: 2005/06 to 2008/09. International Journal of Agricultural Sustainability, Volume 9 (1): 232-247. doi.org/10.3763/ijas.2010.0567.
Druilhe, Z., and J. Barreiro-Hurlé. 2012. Fertilizer subsidies in sub-Saharan Africa (No. 12-04). ESA Working paper. FAO, Rome.
Foley, J.A., N. Ramankutty, K.A. Brauman, E.S. Cassidy, J.S. Gerber, M. Johnston, N.D. Mueller, C. O’Connell, D.K. Ray, P.C. West, C. Balzer, E.M. Bennet, S.R. Carpenter, J. Hill, C. Monfreda, S. Polasky, J. Rockstrom, J. Sheehan, S. Siebert, D. Tilman, DPM Zaks. 2011. Solutions for a cultivated planet. Nature 478: 337-342. Doi: 10.1038/nature10452.
Gautam, M. 2015. Agricultural subsidies: Resurging interest in a perennial debate. Indian Journal of Agricultural Economics, 70 (1): Jan-March.
Gaudin A.C.M, T.N. Tolhurst, A.P. Ker, K. Janovicek, C. Tortora, R.C. Martin, et al. (2015) Increasing Crop Diversity Mitigates Weather Variations and Improves Yield Stability. PLoS ONE 10(2): e0113261. https://doi.org/10.1371/journal.pone.0113261.
Gebremedhin, B., M. Smale, and J. Pender. 2006. Determinants of Cereal Diversity in villages of Northern Ethiopia. In M. Smale, (ed.), Valuing Crop Biodiversity: On-Farm Genetic Resources and Economic Change. Wallingford, Oxon, UK: CAB International.
Hauggaard-Nielsen, H., B. Jørnsgaard, J. Kinane, J., and E. Jensen, E. 2008. Grain legume–cereal intercropping: The practical application of diversity, competition and facilitation in arable and organic cropping systems. Renewable Agriculture and Food Systems, 23(1), 3-12.
Holden, S.T. 2019. Economics of farm input subsidies in Africa. Annual Review of Resource Economics 11: 501-22.
Holden, S.T., C.B. Barrett, and F.Hagos. 2006. Food-for-work for poverty reduction and the promotion of sustainable land use: can it work? Environment and Development Economics 11 (1) 15-38.
Holden, S. and R. Rodney. 2012. Do fertilizer subsidies crowd out organic manures? The case of Malawi. Agricultural Economics. 43. 303-314. 10.1111/j.1574-0862.2012.00584.x.
Isbell, F., P.R. Adler, N. Eisenhauer, D. Fornara, K. Kimmel, C. Kremen, D.K. Letourneau, M. Liebman, H.W. Polley, S. Quijas, and M. Scherer‐Lorenzen. 2017. Benefits of increasing plant diversity in sustainable agroecosystems. Journal of Ecology 105: 871-879. https://doi.org/10.1111/1365-2745.12789.
Jarvis, D.I, Brown, A.H.D., Cuong, P.H., et al. 2008. A global perspective of the richness and evenness of traditional crop-variety diversity maintained by farming communities. PNAS 105 (14) 5326-5331; https://doi.org/10.1073/pnas.0800607105.
Jayne, T. S., and Rashid, S. 2013. Input subsidy programs in sub-Saharan Africa: a synthesis of recent evidence. Agricultural Economics, 44(6), 547-562.
Jayne, T.S., N.M. Mason, W.J. Burke, and J. Ariga. 2018. Review: Taking stock of Africa’s second-generation agricultural input subsidy programs. Food Policy 75: 1-14.
Kato, T. and M. Greeley. 2016. Agricultural Input Subsidies in Sub-Saharan Africa, In: Development Studies – Past, Present and Future, IDS Bulletin 47: 33-48.
Kozicka, M., E. Gotor, W. Ocimati, T. de Jager, E. Kikulwe, J. C.J. Groot. 2020. Responding to future regime shifts with agrobiodiversity: A multi-level perspective on small-scale farming in Uganda. Agricultural Systems 183, 102964. https://doi.org/10.1016/j.agsy.2020.102864.
Lin B. 2011. Resilience in agriculture through crop diversification: Adaptive management for environmental change. Bioscience 61: 183–193.
Magurran A. E. 2004. Measuring Biological Diversity. Blackwell, Oxford, UK.
Mary, S. 2013 ‘Assessing the Impacts of Pillar 1 and 2 Subsidies on TFP in French Crop Farms’, Journal of Agricultural Economics, 64(1), pp. 133–144. doi: 10.1111/j.1477-9552.2012.00365.x.
Meng, E.C.H., J.E. Taylor and S.B. Brush. 1998. Implications for the conservation of wheat landraces in Turkey from a household model of varietal choice. In Smale, M. (ed.) Farmers, Genebanks and Crop Breeding. Kluwer Academic Publishers, Boston, MA, pp. 127-143.
Michler, J.D., and A. L. Josephson. 2017. To specialize or diversify: Agricultural diversity and poverty dynamics in Ethiopia. World Development 89: 214-226.
Morgan, S.N., N.M Mason, N. K. Levine, and O. Zulu-Mbata. 2019. Dis-incentivizing sustainable intensification? The case of Zambia’s maize-fertilizer subsidy program. World Development 122 (2019) 54–69.
Nagarajan, L., M. Smale, and P. Glewwe 2007. Determinants of millet diversity at the household-farm and village-community levels in the drylands of India: the role of local seed systems. Agricultural Economics 36 (2), 157–167.
Newey, W. K. (1987) ‘Efficient estimation of limited dependent variable models with endogenous explanatory variables’, Journal of Econometrics, 36(3), pp. 231–250. doi: https://doi.org/10.1016/0304-4076(87)90001-7.
Nguyen, H.Q. 2017. Analyzing the economies of crop diversification in rural Vietnam using an input distance function. Agricultural Systems 153 (May): 148-156.
Omer, O., U. Pascual, N.P. Russell. 2007. Biodiversity conservation and productivity in intensive agricultural systems. Journal of Agricultural Economics 58(2): 308-29.
Pereira, H.M., L.M. Navarro, I.S. Martins. 2012. Global biodiversity change: the bad, the good, and the unknown. Annu. Rev. Environ. Resourc. 37: 25-50.
Pingali, P. 2017. The Green Revolution and Crop Diversity (Chapter 12). In D. Hunter, L. Guarino, C. Spillane, and P. McKeown (Eds.). Handbook of Agricultural Biodiversity. New York: Routledge.
Raseduzzaman, M., and E. S. Jensen. 2017. Does intercropping enhance yield stability in arable crop production? A meta-analysis. European Journal of Agronomy 91: 25-33. https://doi.org/10.1016/j.eja.2017.09.009.
Redlich, S, E.A. Martin, and I. Steffan‐Dewenter. 2018. Landscape‐level crop diversity benefits biological pest control. Journal of Applied Ecology 55: 2419– 2428. https://doi.org/10.1111/1365-2664.13126.
Renard, D., and D. Tilman. 2019.. National food production stabilized by crop diversity. Nature 571, 257–260 (2019). https://doi.org/10.1038/s41586-019-1316-y.
Smale, M. E. Meng, J.P. Brennan, R. Hu. 2003. Determinants of Spatial Diversity in Modern Wheat: Examples from Australia and China. Agricultural Economics 28: 13-26.
Smale, M. (ed). 2006. Valuing Crop Biodiversity: On-Farm Genetic Resources and Economic Change. Wallingford, Oxon, UK: CAB International.
Smale, M., Thériault, V. and Mason, N. M. (2020) ‘Does subsidizing fertilizer contribute to the diet quality of farm women? Evidence from rural Mali’, Food Security. Food Security, 12(6), pp. 1407–1424. doi: 10.1007/s12571-020-01097-w.
Smith, R. J. and Blundell, R. W. (1986) ‘An Exogeneity Test for the Simultaneous Equation Tobit Model’, Econometrica, 54(3), pp. 679–685.
Sogoba, B., B. Traoré, A. Safia, O. B. Samaké, G. Dembélé, S. Diallo, R. Kaboré, G. B. Benié, R. B. Zougmoré, and K. Goïta. On-Farm Evaluation on Yield and Economic Performance of Cereal-Cowpea Intercropping to Support the Smallholder Farming System in the Soudano-Sahelian Zone of Mali. 2020. Agriculture 10, 214; doi:10.3390/agriculture10060214.
Van Dusen, M., and J. Taylor. 2005. Missing markets and crop diversity: Evidence from Mexico. Environment and Development Economics, 10(4), 513-531. doi:10.1017/S1355770X05002317
Wanzala-Mlobela, M., P. Fuentes, S. Mkumbwa, S. 2013. Practices and policy options for the improved design and implementation of fertilizer subsidy programs in sub-Saharan Africa. NEPAD Agency Policy Study, A joint publication by the NEPAD Planning and Coordinating Agency (NPCA), the United Nations Food and Agriculture Organization, and the International Fertiliser and Development Centre (IFDC).
Wooldridge, J. M. 2002. Applications of Generalized Method of Moments Estimation.
Zoundi , J.S., A. Lalba, J.-P. Tiendrébéogo, and D. Bambara. 2007. Systèmes de culture améliorés a base de niébé (Vigna unguiculata (L.) Walp) pour une meilleure gestion de la sécurite alimentaire et des ressources naturelles en zone semi-aride du Burkina Faso. Tropicultura 25 (3): 87-96.
Table 1. Variable definition and summary statistics
Variable |
Mean |
Std. Dev. |
Min |
Max |
|
Dependent variables |
|||||
Menhinick |
Menhinick's diversity index=count of all crops grown/sqrt(total crop area) |
0.792 |
0.527 |
0.064 |
6.708 |
Shannon |
Shannon's diversity index=-sum(crop area shares*ln(crop area shares)) |
0.784 |
0.519 |
0 |
2.10 |
cowpea |
share of cowpea plots |
0.04 |
0.088 |
0 |
1 |
intercowpea |
share of cowpea plots in intercropping |
0.015 |
0.056 |
0 |
0.471 |
Endogenous regressor |
|||||
subsidy |
total amount of subsidized fertilizer (in Kgs) |
268.188 |
813.678 |
0 |
10500 |
Independent variables |
|||||
organic |
hare of plots fertilized with organic fertilizer |
.196 |
.291 |
0 |
1 |
pesticides |
share of plots treated with pesticides |
.127 |
.124 |
0 |
.63 |
dist_road |
distance from household to tarmac road (km) |
12.684 |
12.37 |
0 |
63 |
dist_admctr |
distance from household to administrative capital of the region (km) |
114.182 |
70.789 |
0 |
321 |
popdensity |
2009 population density |
584.386 |
1942.071 |
0 |
11650 |
hhsize |
average household size |
11.656 |
5.402 |
3 |
84 |
educ |
share of plots managers who went to school |
0.235 |
0.195 |
0 |
0.909 |
year2 |
dummy (=1 if year=2017, 0 else) |
0.488 |
0.5 |
0 |
1 |
temperature |
annual mean temperature (deg C) |
276.08 |
7.468 |
258 |
300 |
rainfall |
12-month total rainfall (mm) |
611.687 |
195.625 |
183 |
1050 |
a800mm |
dummy(=1 if zone above the 800 mm isohyet, 0 else) |
.335 |
.472 |
0 |
1 |
bw400_800mm |
dummy(=1 if zone between isohyets 400 and 800 mm, 0 else) |
.616 |
.486 |
0 |
1 |
Instrumental variables |
|||||
fertilizer |
amount of commercial fertilizer (in Kgs) |
5801.924 |
42402.025 |
0 |
1312550 |
DNA |
dummy (=1 if supervised by DNA, 0 else) |
0.493 |
0.5 |
0 |
1 |
ON |
dummy (=1 if supervised by ON), 0 else) |
0.06 |
0.238 |
0 |
1 |
CMDT |
dummy (=1 if supervised by CMDT, 0 else) |
0.193 |
0.395 |
0 |
1 |
OTHERS |
dummy (=1 if supervised by other services or NGOs, 0 else) |
0.255 |
0.436 |
0 |
1 |
Source: Authors, based on LSMS 2014-15 and 2017-18.
Note: Variables measured at SE (“village”) scale.
Table 2. IV-Tobit First-stage regression
subsidy |
Coef. |
Std. Err. |
t |
P>t |
[95% Conf. |
Interval] |
Sig |
comfertilizer |
0.016 |
0.004 |
1.540 |
0.082 |
-0.019 |
0.002 |
* |
DNA |
0.045 |
0.042 |
1.090 |
0.028 |
-0.036 |
0.127 |
** |
ON |
0.297 |
0.087 |
3.400 |
0.001 |
0.126 |
0.468 |
*** |
CMDT |
0.612 |
0.067 |
9.100 |
0.000 |
0.480 |
0.744 |
*** |
organic |
-0.251 |
0.103 |
-2.440 |
0.015 |
-0.453 |
-0.049 |
** |
pesticides |
0.355 |
0.079 |
4.520 |
0.000 |
0.201 |
0.509 |
*** |
dist_road |
-0.319 |
0.168 |
-1.900 |
0.058 |
-0.648 |
0.011 |
* |
dist_admctr |
-0.018 |
0.032 |
-0.580 |
0.565 |
-0.080 |
0.044 |
|
temperature |
-0.724 |
0.365 |
-1.980 |
0.047 |
-1.439 |
-0.008 |
** |
rainfall |
0.134 |
0.055 |
2.440 |
0.015 |
0.026 |
0.242 |
** |
popdensity |
-0.108 |
0.038 |
-2.840 |
0.005 |
-0.182 |
-0.033 |
|
a800mm |
0.134 |
0.141 |
0.950 |
0.343 |
-0.143 |
0.410 |
|
bw400_800mm |
-0.055 |
0.115 |
-0.470 |
0.636 |
-0.281 |
0.171 |
|
hhsize |
0.010 |
0.004 |
2.520 |
0.012 |
0.002 |
0.018 |
* |
educ |
0.070 |
0.114 |
0.620 |
0.538 |
-0.154 |
0.295 |
|
year2 |
-0.035 |
0.046 |
-0.770 |
0.442 |
-0.126 |
0.055 |
|
_cons |
1.957 |
1.079 |
1.810 |
0.070 |
-0.158 |
4.073 |
*** |
F-statistic |
25.460 |
Prob>F |
0.000 |
||||
N |
|
1464 |
|
|
|
|
|
Source: Authors, based on LSMS 2014-15 and 2017-18. *** p<.01, ** p<.05, * p<.1
Table 3. IV-Tobit second stage-regression
|
menhinick |
shannon |
cowpea |
intercowpea |
subsidy |
-.028** |
.35*** |
-.004* |
0.039 |
organic |
0.037 |
.417*** |
.071*** |
.22*** |
pesticides |
-.252*** |
-.413*** |
-.1*** |
-.165*** |
dist_road |
-.148* |
-0.064 |
0.036 |
-0.089 |
dist_admctr |
.04*** |
.136*** |
.006** |
.015** |
temperature |
.734*** |
1.443*** |
-0.138 |
-.403** |
rainfall |
.087*** |
.201*** |
.01** |
-0.002 |
popdensity |
-0.005 |
-.047*** |
-0.001* |
-.013* |
a800mm |
-0.053 |
-.212** |
-0.021 |
0.005 |
bw400_800mm |
-.125** |
-0.036 |
-0.006 |
0.104 |
hhsize |
-.006*** |
.007** |
.002** |
0.002 |
educ |
.234*** |
-0.007 |
.06** |
0.04 |
Year2 |
-.247*** |
.053* |
-0.015 |
-.092*** |
_cons |
-1.533*** |
-4.697*** |
0.255 |
0.816 |
Wald test of exogeneity χ2 |
0.535** |
55.73*** |
1.91** |
.64* |
N |
1464 |
1464 |
1464 |
1464 |
Source: Authors, based on LSMS 2014-15 and 2017-18. *** p<.01, ** p<.05, * p<.1
Table 4. GMM regression results
|
Menhinick |
Shannon |
Cowpea |
Intercowpea |
Menhinick |
1.494** |
1.411* |
||
shannon |
6.002** |
0.273 |
||
subsidy |
-0.246** |
0.021 |
-0.104* |
-0.256 |
organic |
-0.717** |
-0.301* |
1.967 |
4.642** |
pesticides |
-0.210** |
-0.168*** |
-0.001 |
-0.912 |
dist_road |
-0.235** |
-0.076 |
1.421* |
-0.192 |
dist_admctr |
0.054** |
0.067*** |
-0.096 |
0.398 |
temperature |
-0.213 |
0.085 |
-3.343** |
-3.152*** |
rainfall |
0.307*** |
0.158** |
0.362 |
1.360** |
popdensity |
-0.022** |
-0.002 |
-0.002 |
-0.033** |
a800mm |
-0.310** |
-0.300*** |
1.648 |
-0.015 |
bw400_800mm |
-0.557** |
-0.310** |
1.727* |
0.982 |
hhsize |
-0.005 |
0.003* |
0.035 |
0.027 |
educ |
0.138 |
-0.084 |
0.358 |
0.164 |
year2 |
-0.499* |
-0.497** |
1.622 |
-5.14 |
_cons |
-0.969 |
0.4 |
|
|
Hansen's J |
chi2(10) = 19.87 (p = 0.0705) |
|||
N |
1464 |
1464 |
1464 |
1464 |
Source: Authors, based on LSMS 2014-15 and 2017-18. *** p<.01, ** p<.05, * p<.1
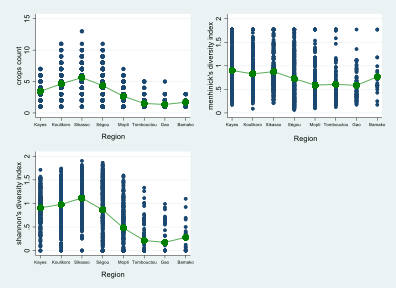
Source: Authors
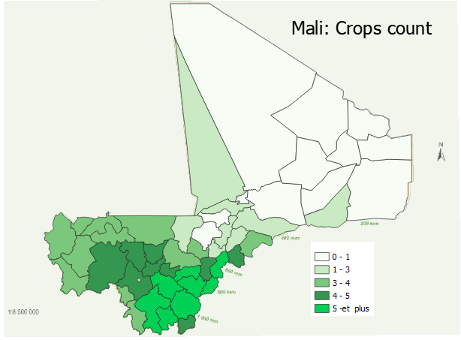
Source: Authors, based on LSMS 2014-15 and 2017-18.
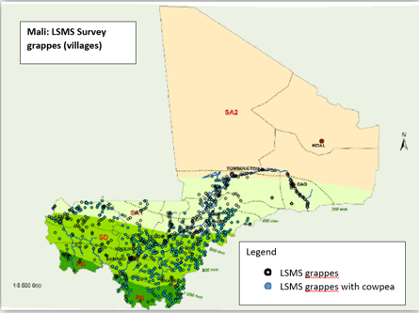
Source: Authors, based on LSMS 2014-15 and 2017-18.